Role of Machine Learning in Anticipating Adverse Drug Reactions: Implications for Patient Safety in Pharmacy Practice
DOI:
https://doi.org/10.71143/nv3qs952Keywords:
Adverse Drug Reactions, Machine Learning, Patient Safety, Pharmacovigilance, Predictive Analytics, Pharmacy Practice, Deep Learning, Drug SafetyAbstract
Adverse drug reactions (ADRs) are a major concern in the field of pharmacology, significantly impacting patient safety and healthcare costs. As drug interactions become more complex, traditional methods for predicting ADRs often fall short. However, the application of machine learning (ML) techniques presents a promising solution for improving the prediction and management of these reactions, offering valuable insights into their underlying mechanisms. By leveraging ML, healthcare professionals can better anticipate ADRs, leading to safer medication practices and enhanced patient care. This innovative approach not only helps in identifying potential risks associated with medications but also supports proactive measures to mitigate these risks, ultimately contributing to improved health outcomes for patients. This paper explores the role of ML in anticipating ADRs by analysing diverse datasets, including clinical records, drug properties, and patient demographics. We discuss various ML models, such as deep learning and ensemble methods, that have shown efficacy in identifying potential ADRs before they manifest clinically. By leveraging large volumes of health-related data, these models can improve the accuracy of predictions, facilitate timely interventions, and ultimately enhance patient safety. This research underscores the necessity for ongoing collaboration between data scientists and healthcare professionals to optimize the application of ML in real-world settings.
Downloads
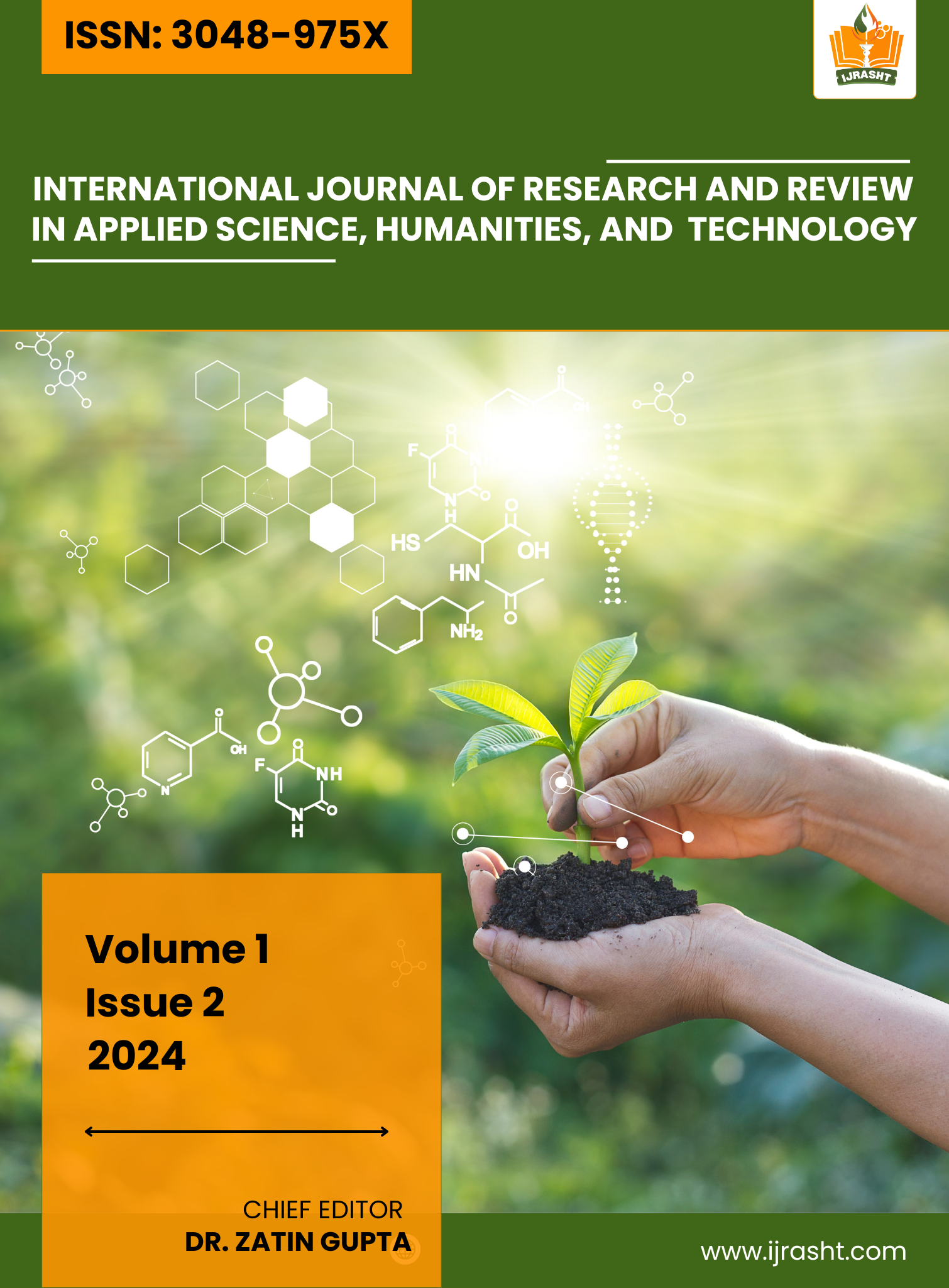
Downloads
Published
Issue
Section
License
Copyright (c) 2024 International Journal of Research and Review in Applied Science, Humanities, and Technology

This work is licensed under a Creative Commons Attribution-NonCommercial 4.0 International License.